Define Data Visualization and Mention its Importance
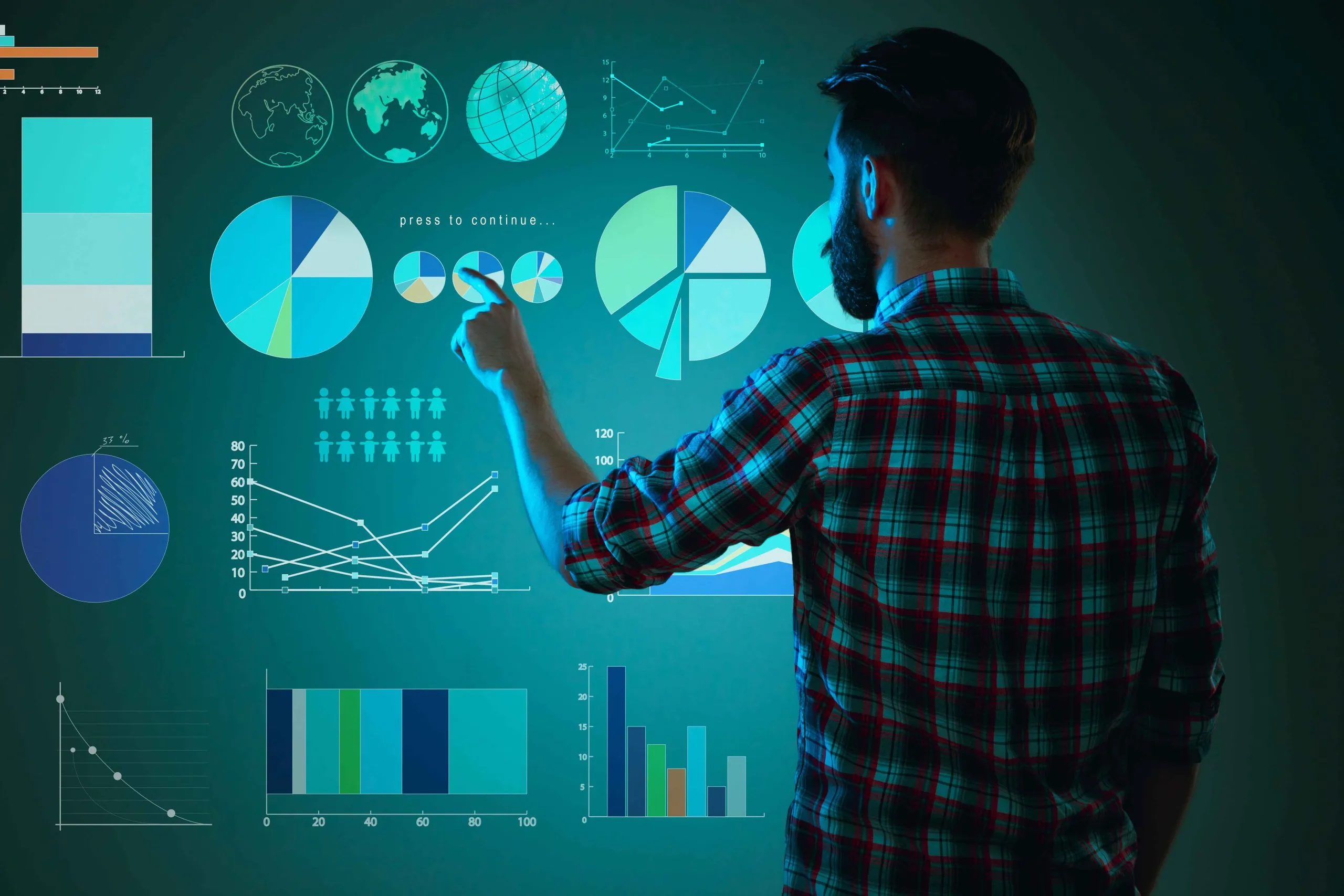
Data visualization is the graphical representation of data using charts, graphs, maps, and other visual elements. It translates complex datasets into easily understandable and visually compelling formats, allowing patterns, trends, and insights to be quickly identified and comprehended.
In today’s data-driven world, data visualization services are essential for several reasons:
- Clarity and Understanding: With the explosion of data, data visualization helps present information clearly and intuitively. It lets individuals grasp vast amounts of data at a glance, making complex data more accessible to a broader audience.
- Insight Discovery: Visual representations of data make it easier to identify patterns, trends, and correlations that may not be apparent in raw data. This leads to valuable insights and helps in making informed decisions.
- Communication and Storytelling: Data visualization enhances communication by turning numbers into visual narratives. It enables data-driven storytelling, allowing analysts and decision-makers to present findings, trends, and outcomes compellingly and persuasively.
- Speed and Efficiency: Visualizations enable quick data analysis. Users can quickly identify outliers, understand data distributions, and draw conclusions more efficiently, leading to faster decision-making processes.
- Data Exploration: Visualization tools support interactive data exploration, enabling users to filter, drill down, and interact with the data to gain deeper insights and answer specific questions.
- Enhanced Business Intelligence: Data visualization is a fundamental component of business intelligence (BI) systems, empowering organizations to monitor performance, track KPIs, and respond to changing trends promptly.
- Engagement and Memory Retention: Visual representations are more engaging and memorable than plain text or numerical data. They leave a lasting impression, aiding in better retention and recall of information.
- Identification of Anomalies and Outliers: Visualization can help detect anomalies and outliers in data, which are crucial in fraud detection, quality control, and error identification.
- Collaboration and Data Sharing: Visualizations facilitate collaborative decision-making by giving team members a common understanding of data. They can be easily shared across departments and stakeholders.
- Data-Backed Storytelling: In fields like journalism and marketing, data visualization enables journalists and advertisers to use data to back their narratives and make data-supported arguments.
In conclusion, data visualization is vital in making data meaningful, actionable, and accessible to many users. It is a powerful tool for organizations and individuals to explore data, gain insights, and make informed decisions in today’s data-driven and information-rich environment.
Benefits of Using Data Visualization in Business Decision-Making
Using data visualization in business decision-making processes offers numerous key benefits:
- Clear Insights: Data visualization transforms complex datasets into visually intuitive charts, graphs, and dashboards, enabling decision-makers to grasp insights and trends quickly.
- Improved Decision-Making: Visual data representations facilitate better understanding and analysis, leading to more informed and data-driven decisions.
- Quick Identifications: Visualizations help in spotting patterns, outliers, and correlations promptly, allowing businesses to address emerging issues promptly.
- Enhanced Communication: Data visualizations provide a common ground for sharing and discussing data across teams and departments, fostering better communication and alignment.
- Data Storytelling: Visualizations support data storytelling, enabling decision-makers to present findings and recommendations compellingly and persuasively.
- Interactivity and Exploration: Interactive data visualizations allow users to explore data from various angles, filter information, and drill down to details for deeper insights.
- Real-Time Monitoring: Visual dashboards and reports enable real-time monitoring of key performance indicators (KPIs), helping businesses stay agile and responsive to changing conditions.
- Predictive Analysis: Visualizations aid in identifying trends and patterns that can be used for predictive analysis, empowering businesses to anticipate future outcomes.
- Engagement and Buy-In: Visual representations captivate stakeholders and increase engagement, leading to better buy-in and support for data-driven initiatives.
- Identifying Opportunities and Risks: Data visualization outsourcing services enable businesses to identify growth opportunities and potential risks, guiding strategic planning and risk management efforts.
- Efficient Resource Allocation: With more precise insights, organizations can allocate resources more efficiently, optimizing budgets and improving operational efficiency.
- Competitive Advantage: Leveraging data visualization as a decision-making tool can provide a competitive advantage by enabling businesses to respond faster to market changes and customer needs.
- Better Customer Understanding: Data visualizations allow businesses to understand customer behavior, preferences, and feedback, leading to improved customer satisfaction and loyalty.
- Alignment with Business Goals: Visualizing key metrics and performance indicators ensures that decision-making aligns with the overall business objectives and strategies.
- Error Detection and Quality Control: Visual representations facilitate spotting errors and inconsistencies in data, improving data quality and reliability.
In summary, data visualization empowers businesses to leverage data effectively, derive actionable insights, and make well-informed decisions, ultimately contributing to enhanced efficiency, profitability, and market competitiveness.
Different Data Visualizations with Respective Use Cases
There are various data visualizations, each with unique characteristics and best-suited use cases. Here are some common types and their respective applications:
- Bar Charts
Use Case: Bar charts are ideal for comparing categorical data or displaying discrete values. They are suitable for visualizing data like sales by region, the number of customers in different age groups, or comparing performance metrics across other teams.
- Line Charts
Use Case: Line charts effectively show trends and changes over time. They are often used to visualize time-series data, such as stock prices, temperature fluctuations, or website traffic over months or years.
- Pie Charts
Use Case: Pie charts represent parts of a whole, where each slice represents a proportion of the total. They are suitable for visualizing market share, survey responses, or budget allocations.
- Scatter Plots
Use Case: Scatter plots display the relationship between two numerical variables. They are often used in scientific and engineering applications to show correlations, clusters, or outliers in data.
- Heat Maps
Use Case: Heat maps are useful for visualizing large datasets and identifying patterns or trends. They are commonly used in geographic analysis, showing population density, weather patterns, or customer locations.
- Histograms
Use Case: Histograms display the distribution of continuous data and identify frequency or probability. They help analyze data like exam scores, product ratings, or response times.
- Area Charts
Use Case: Area charts are similar to line charts but are filled with color to show the area under the lines. They are suitable for illustrating cumulative data over time, such as tracking website visits or revenue growth.
- Bubble Charts
Use Case: Bubble charts visualize three dimensions of data, with the size of the bubbles representing the third variable. They are often used to show the relationship between three factors: GDP, population, and countries’ carbon emissions.
- Gantt Charts
Use Case: Gantt charts are commonly used in project management to display the schedule of tasks, their dependencies, and progress over time.
- Tree Maps
Use Case: Tree maps are useful for visualizing hierarchical data and representing proportions within each level. They are used in disk space analysis, portfolio allocation, or organizational hierarchies.
These are just a few examples of the many data visualization types available. Choosing the right visualization depends on the data’s nature, the story you want to convey, and the insights you aim to gain.
Best Data Visualization Tools for Business Analytics
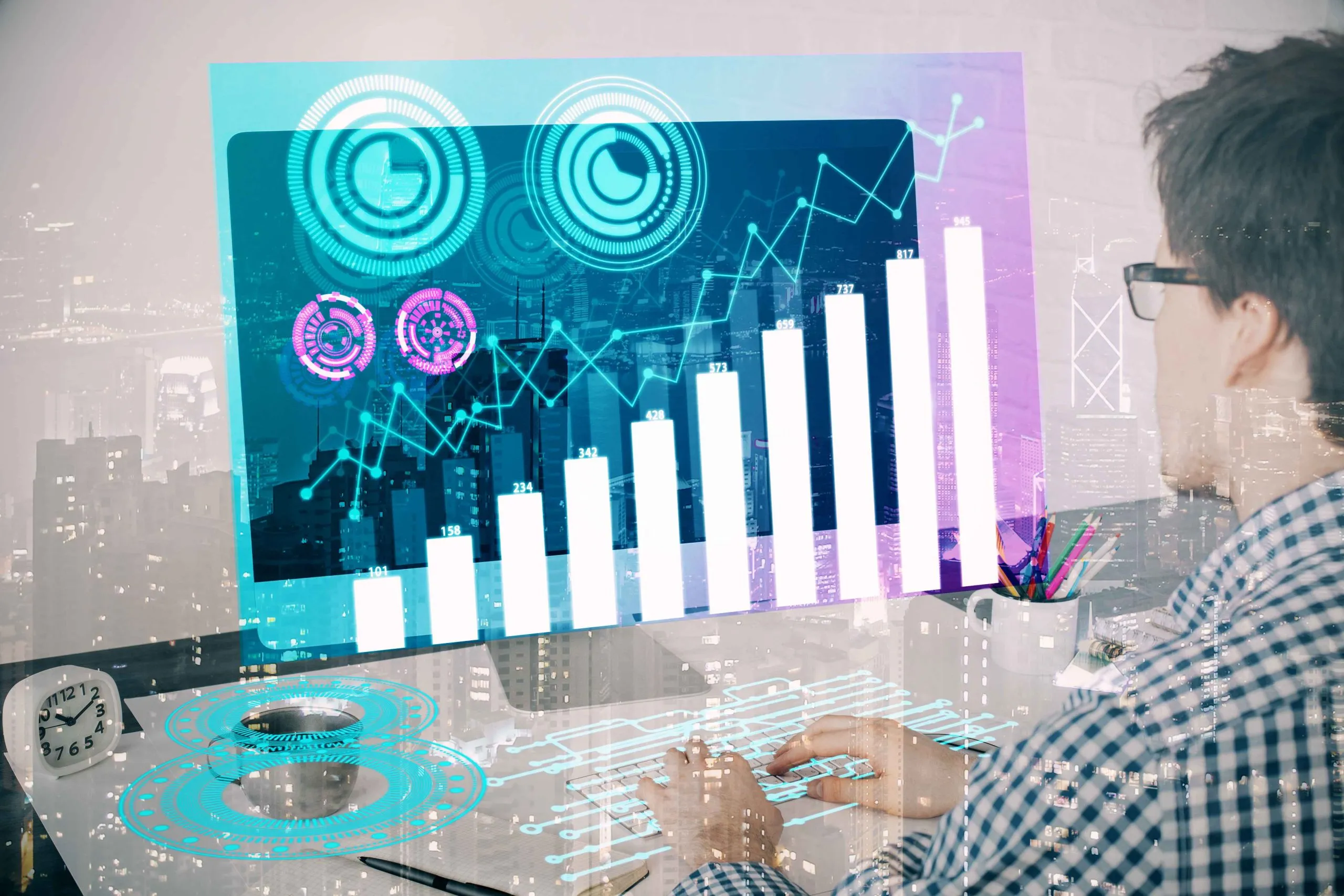
Several powerful data visualization tools are available for business analytics, each offering unique features and capabilities. Some of the best and most widely used data visualization tools are:
- Tableau: Tableau is a leading data visualization and business intelligence platform known for its user-friendly interface and robust features. It allows users to create interactive dashboards, charts, and graphs effortlessly, enabling data exploration and analysis without complex coding.
- Microsoft Power BI: Power BI is a popular data visualization tool that seamlessly integrates with other Microsoft products. It offers a wide range of visualization options, real-time data dashboards, and interactive reports, making it an excellent choice for businesses heavily reliant on Microsoft’s ecosystem.
- Qlik Sense: Qlik Sense provides self-service data visualization and exploration capabilities, allowing users to drag and drop data to create interactive visualizations and reports. Its associative data model allows for intuitive data exploration, making it easy to identify relationships and insights.
- Google Data Studio: Google Data Studio is a free cloud-based data visualization tool that integrates seamlessly with various Google products, including Google Analytics and Google Sheets. It enables users to create customizable and interactive reports and dashboards with real-time data.
- Domo: Domo is a cloud-based business intelligence platform combining data visualization, integration, and collaboration features. It is designed to help businesses consolidate data from multiple sources and create intuitive dashboards for data analysis and decision-making.
- Sisense: Sisense is known for its ability to handle large and complex datasets and provide fast and powerful data visualization and analytics solutions. It is suitable for businesses that require robust and scalable data visualization capabilities.
- Looker: Looker is a data visualization and business intelligence platform emphasizing data exploration and collaboration. It allows users to create custom dashboards and reports and facilitates data sharing across the organization.
- Plotly: Plotly is an open-source data visualization library that offers a range of visualization options, including interactive charts, plots, and maps. It is popular among data scientists and developers for creating data visualizations in Python, R, and JavaScript.
- Zoho Analytics: Zoho Analytics provides comprehensive data visualization and business intelligence tools for data analysis, reporting, and dashboard creation. It suits small to medium-sized businesses looking for a user-friendly analytics solution.
- Highcharts: Highcharts is a popular JavaScript-based data visualization library that offers a wide range of charts and interactive features. It is often used for embedding dynamic and responsive data visualizations into web applications.
The best data visualization tool for business analytics depends on factors such as the organization’s specific needs, data sources, budget, and technical expertise available within the team. Each tool has its strengths and can significantly enhance data-driven decision-making processes.
Tableau Vs. Power BI for Data Visualization
Tableau and Power BI are two leading data visualization tools with their own set of advantages and limitations. Let’s compare the pros and cons of each:
Tableau
Pros:
- User-Friendly Interface: Tableau offers an intuitive drag-and-drop interface, making it easy for non-technical users to create visualizations without extensive coding knowledge.
- Robust Data Connectivity: It supports many data sources, including databases, cloud storage, and web connectors, allowing seamless integration and analysis of diverse datasets.
- Advanced Analytics: Tableau provides powerful analytics capabilities, including forecasting, statistical functions, and complex calculations, enabling deeper data exploration.
- Highly Interactive Dashboards: Tableau allows users to create interactive and dynamic dashboards that facilitate real-time data exploration and insights.
- Large Online Community: There is a vast and active Tableau user community, providing extensive resources, tutorials, and support for troubleshooting.
Cons:
- Pricing: Tableau is considered relatively more expensive than some other data visualization tools, making it less accessible for smaller organizations or individual users.
- Learning Curve: While the interface is user-friendly, mastering Tableau’s advanced features may require some learning for new users.
- Limited Collaboration Features: Collaborative capabilities in Tableau are less extensive than other tools, which may affect team collaboration on larger projects.
Power BI:
Pros:
- Integration with Microsoft Ecosystem: Power BI seamlessly integrates with other Microsoft products, such as Excel, Azure, and SharePoint, making it an excellent choice for organizations heavily reliant on Microsoft technologies.
- Affordability: Power BI offers cost-effective pricing plans, including a free version with limited features, making it more accessible to small businesses and individuals.
- Natural Language Query: Power BI’s “Q&A” feature allows users to use natural language queries to retrieve data, making exploring data without specific technical knowledge easier.
- Strong Data Modeling: It has robust data modeling capabilities, which is beneficial for data preparation and transformation tasks.
- Strong Collaboration Features: Power BI allows for extensive collaboration and sharing of reports and dashboards with colleagues and stakeholders.
Cons:
- Limited Advanced Analytics: While Power BI offers some advanced analytics features, Tableau is more comprehensive regarding statistical analysis and forecasting capabilities.
- Performance on Large Datasets: Power BI may experience performance issues when handling large and complex datasets compared to Tableau.
- Customization Options: Power BI offers fewer customization options for visualizations than Tableau.
Ultimately, the choice between Tableau and Power BI depends on the organization’s specific needs, budget, existing technology stack, and the level of advanced analytics required. Both tools are powerful and widely used, and businesses should evaluate their individual requirements to make an informed decision.
Key Differences Between Static and Interactive Data Visualizations
Static Data Visualizations:
Static data visualizations are non-interactive visual representations of data that do not allow users to manipulate or explore the data directly. They are pre-generated and remain fixed, displaying the same information regardless of user input. Common examples of static visualizations include printed charts, infographics, and images.
Key Characteristics:
- Fixed Content: Static visualizations have predetermined content that cannot be changed or updated dynamically.
Limited User Engagement: Users can only observe the data presented without the ability to interact or drill down for more details.
- Easy to Share: Static visualizations are easy to share as images or documents, making them suitable for presentations and reports.
- Low Technical Complexity: Creating static visualizations typically requires basic data visualization skills and tools like Microsoft Excel or PowerPoint.
When to Use Static Data Visualizations:
Static data visualizations are useful in scenarios where:
- The data and insights are straightforward and don’t require further exploration.
- The audience is passive and doesn’t need to interact with the data directly.
- The visualization is intended for print media, reports, or presentation slides.
- The data will likely stay the same, and periodic updates are sufficient.
Interactive Data Visualizations:
Interactive data visualizations allow users to actively engage with the data by manipulating and exploring it directly. Users can interact with the visualization elements, apply filters, drill down into details, and even change the data displayed dynamically. These visualizations are often web-based or embedded in applications.
Key Characteristics:
- User Engagement: Interactive visualizations encourage user engagement, enabling them to explore data, uncover insights, and answer specific questions.
- Dynamic Updates: Data in interactive visualizations can be updated in real-time, ensuring the latest information is available to users.
- Exploratory Nature: Interactive visualizations are ideal for data exploration and hypothesis testing, allowing users to discover patterns and relationships.
- Higher Technical Complexity: Developing interactive visualizations may require more advanced skills using tools like Tableau, Power BI, D3.js, or other programming languages.
When to Use Interactive Data Visualizations:
Interactive data visualizations are beneficial when:
- Users need to explore complex and multifaceted data to gain deeper insights.
- The data is dynamic and requires real-time updates to reflect changes.
- Users must filter data based on specific criteria or compare different data subsets.
- The visualization is intended for web applications, dashboards, or self-service analytics.
In summary, the choice between static and interactive data visualizations depends on the purpose of the visualization, the level of engagement required, and the target audience’s needs. Static visualizations are suitable for presenting simple and fixed information, while interactive visualizations enable deeper exploration and data-driven decision-making.
Latest Trends and Innovations in Data Visualization
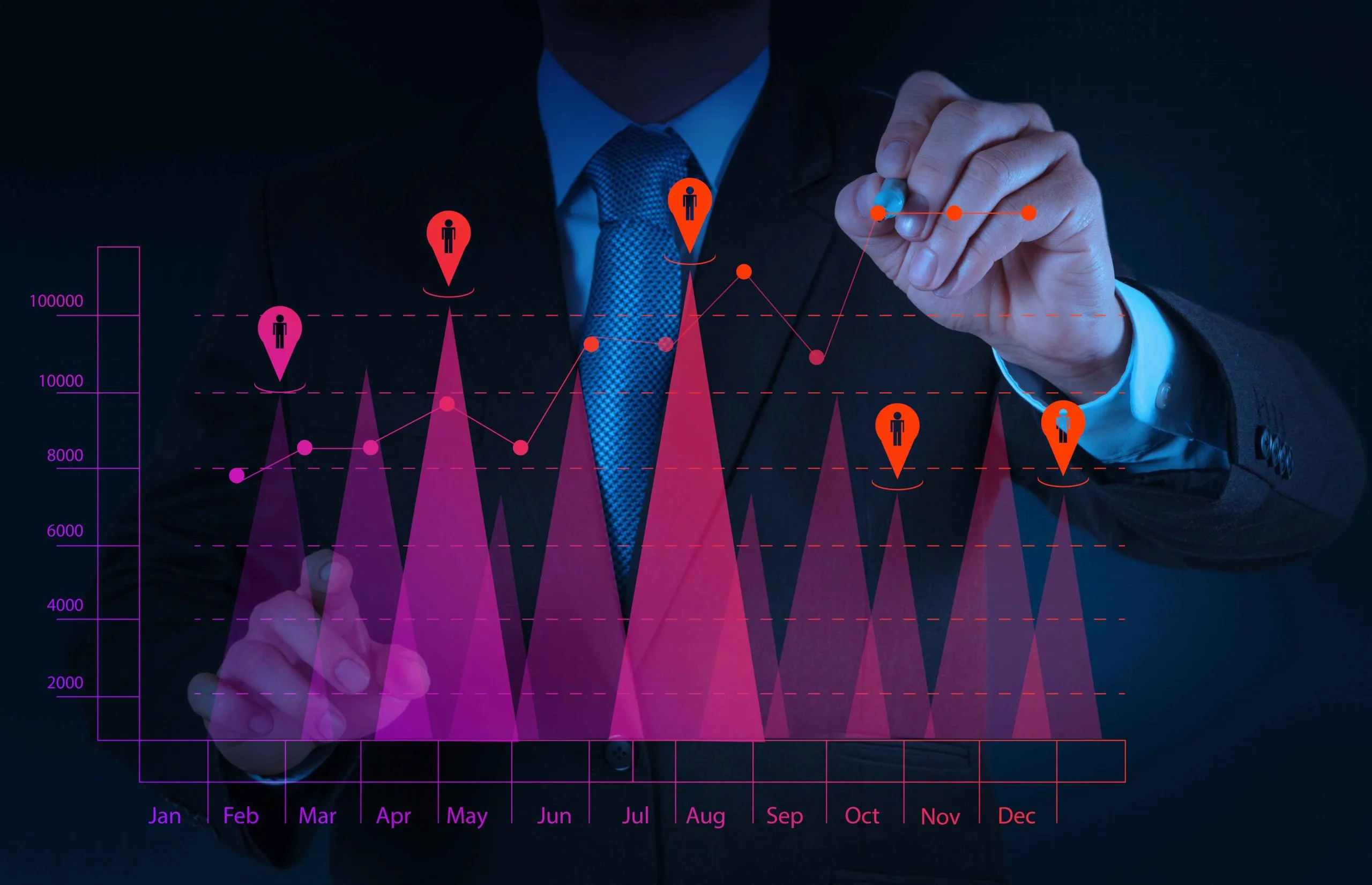
As of my last update in September 2021, the field of data visualization continues to evolve rapidly with new trends and innovations emerging. While I don’t have access to real-time information, some of the latest trends and innovations in data visualization technologies up to that time were:
- Augmented Reality (AR) and Virtual Reality (VR) Visualization: Integrating data visualization with AR and VR technologies allows users to interact with data in immersive and three-dimensional environments, providing a more engaging and insightful experience.
- Natural Language Processing (NLP) and Data Storytelling: Advancements in NLP enable data visualization tools to interpret natural language queries, allowing users to interact with data using voice commands and receive visualizations as answers. Data storytelling techniques are also becoming more prevalent, making visualizations more narrative-driven and compelling.
- Big Data Visualization: As organizations deal with ever-growing data volumes, visualization tools adapt to handle big data more efficiently. Innovations in data compression, caching, and parallel processing enhance the performance of visualizations on massive datasets.
- Explainable AI and Machine Learning Visualization: With the increasing adoption of AI and machine learning models, there is a growing need for explainable AI and interpretable visualizations to understand and communicate complex model outputs and predictions.
- Real-Time Data Visualization: The demand for real-time insights has led to the development of visualization tools that can process and display data in real-time, enabling organizations to make timely decisions based on up-to-date information.
- Data Collaboration and Sharing: Collaborative data visualization platforms allow multiple users to collaborate on the same visualization simultaneously, fostering teamwork and knowledge sharing across departments.
- Automated Insights and Anomaly Detection: Visualization tools incorporate automated insights and anomaly detection algorithms, helping users quickly identify patterns, outliers, and trends in data without manual exploration.
- Geospatial Visualization and Mapping: Geospatial visualization capabilities have improved, enabling more accurate and interactive data mapping to visualize spatial relationships and geographic trends.
- Responsive and Mobile-Friendly Visualizations: With the increasing use of mobile devices, data visualization technologies optimize visualizations for responsive design, ensuring they are accessible and user-friendly across different screen sizes.
- Data Governance and Security: As data privacy and security become paramount, visualization tools enhance data governance features to ensure compliance with regulations and protect sensitive information.